Tuesday, October 22, 2024
Our AI models are tiny, but training them is complex and costly
This chart captures the intensity of our cloud compute usage over two weeks in September. Each colored bar serves as a reminder of the complexity and cost required to build truly intelligent camera systems.
Our AI may be tiny, but our AI factory is anything but simple. Every day, it handles thousands of tasks—training models on over 30 million images and videos, optimizing for different platforms, and rigorously fine-tuning, testing, and validating each one. All of this happens in the data center, where costs quickly skyrocket when pushing the state-of-the-art in AI.
We handle the heavy lifting of AI development, so you can focus on what matters most—delivering the best product to your customers. We operate the AI factory so you can enjoy cutting-edge technology, without the hassle and cost of managing it yourself.
Want to see results? Try our online demo.
Monday, October 7, 2024
iPod Inventor and Nest Founder Tony Fadell Backs Plumerai’s Tiny AI, Hailing It as a Massive Market Disruption Democratizing AI Technology Starting with Smart Home Cameras
Plumerai Announces Partnership with Chamberlain Group, the Largest Manufacturer of Automated Access Solutions found in 50+ million homes
Press release
London – October 7, 2024 - Plumerai™, a pioneer in on-device AI solutions since 2018, today announced a major partnership with Chamberlain Group, whose brands include myQ and LiftMaster, marking a significant milestone in the adoption of its Tiny AI technology.
To achieve features like People Detection and Familiar Face Identification, cloud-based AI and, in particular, Large Language Models (LLMs) require vast remote data centers, consume increasing amounts of energy, pose privacy risks, and incur rising costs. Plumerai’s Tiny AI can do all this on the device itself, is cost-effective, chip agnostic, capable of operating on battery-powered devices, doesn’t clog up your bandwidth with huge video uploads, and has minuscule energy requirements. Moreover, it boasts the most accurate on-device Tiny AI on the market and offers end-to-end encryption. Already running locally on millions of smart home cameras, Plumerai’s Tiny AI is making communities safer and lives more convenient, while proving that in AI, smaller can indeed be smarter. Plumerai has gained strong backing from early investor Tony Fadell, Principal at Build Collective along with Dr. Hermann Hauser KBE, Founder of Arm, and LocalGlobe.
“Tiny AI is a paradigm shift in how we approach artificial intelligence,” said Roeland Nusselder, Co-founder and CEO at Plumerai. “This approach allows us to embed powerful AI capabilities directly into smart home devices, enhancing security and privacy in ways that were once considered unfeasible. It’s not about making AI bigger - it’s about making it smarter, more accessible, and more aligned with people’s real-world needs."
Key Features of Plumerai’s Tiny AI on the Edge, No Cloud Required
- Person, Pet, Vehicle, and Package Detection notifications in .7 seconds vs. average smart cam 2-10 seconds. This may sound like an incremental improvement, but anyone who has a smart cam will appreciate this is a game-changer.
- Familiar Face Identification and Stranger Detection deliver accurate notifications while protecting privacy; tag up to 40 “safe” i.e. familiar individuals.
- Multi-Cam Person Tracking (first of its kind!) for comprehensive surveillance.
- Advanced Motion Detection with rapid response time of, on average, .5 seconds which greatly extends battery life.
- Over-the-Air updates for products in the field means faster Go-to-Market.
Plumerai has built the most accurate Tiny AI solution for smart home cameras, trained with over 30 million images and videos. Consistently outperforming competitors in every commercial shootout, Plumerai is the undisputed leader in both accuracy and compute efficiency. Internal test comparisons against a leading smart camera, widely regarded as the most accurate among established smart home players, revealed striking results: leading smart camera’s AI incorrectly identified strangers as household members in 2% of the recorded events, while Plumerai’s Familiar Face Identification made no incorrect identifications.
“Plumerai’s technology gives companies a significant edge in a competitive market, proving that efficient, on-device AI is the future of smart home security. It’s a perfect showcase for Tiny AI’s strengths - privacy, real-time processing, and energy efficiency. I’m excited by Plumerai’s potential to expand into other IoT verticals and redefine edge computing,” said Hermann Hauser, Founder of Arm.
Providing a ready-to-integrate end-to-end solution for AI on the edge, Plumerai is making AI more competitive and a reality for more companies. This breakthrough provides consumers with more options outside the realm of big tech giants like Google, Amazon, Microsoft, and Apple. Unlike other battery powered “smart” cams that send their video feed to the cloud over power-hungry Wi-Fi, Plumerai enables the use of low-power mesh networks to send notifications. With Plumerai, companies can easily change their chips without losing AI features.
“Integrating Plumerai’s Tiny AI into our smart camera lineup has allowed us to offer advanced AI features on affordable, low-power devices. This enables us to provide our customers with smarter, more efficient products that deliver high performance without the need for cloud processing,” said Jishnu Kinwar, VP Advanced Products at Chamberlain Group.
Notably, 11+ million people rely on Chamberlain’s myQ® app daily to access and monitor their homes, communities and businesses, from anywhere.
“Plumerai’s Tiny AI isn’t just an incremental advance – it’s a massive market disruption,” said Tony Fadell, Nest Founder and Build Collective Principal. “While Large Language Models capture headlines, Plumerai is getting commercial traction with its new smaller-is-smarter AI model. Their Tiny AI is faster, cheaper, more accurate, and doesn’t require armies of developers harnessing vast data centers.”
About Plumerai
Plumerai is a pioneering leader in embedded AI solutions, specializing in highly accurate and efficient Tiny AI for smart devices. As the market leader in licensable Tiny AI for smart home cameras, Plumerai powers millions of devices worldwide and is rapidly expanding into video conferencing, smart retail, security, and IoT sectors. Their comprehensive AI suite includes People Detection, Vehicle detection, Animal detection, Familiar Face Identification, and Multi-Cam Person Tacking, all designed to run locally on nearly any camera chip. Headquartered in London and Amsterdam and backed by Tony Fadell, Principal at Build Collective, Dr. Hermann Hauser, Founder of Arm, and LocalGlobe. Plumerai enables developers to add sophisticated AI capabilities to embedded devices, revolutionizing smart technology while prioritizing efficiency and user privacy.
About Build Collective
Build Collective, led by Tony Fadell, is a global investment and advisory firm coaching engineers, scientists, and entrepreneurs working on foundational deep technology. With 200+ startups in its portfolio, Build Collective invests its money and time to help engineers and scientists bring technology out of the lab and into our lives. Supporting companies beyond Silicon Valley, Build Collective’s portfolio is based mainly in the EU and US with some companies in Asia and the Middle East. From tackling food security, sustainability, transportation, energy efficiency, weather, robotics, and disease to empowering small business owners, entrepreneurs, and consumers, the startups in Build Collective’s portfolio are improving our lives and prospects for the future. With no LP’s to report to, the Build Collective team is hands-on and advises for the long-term.
About Chamberlain Group
Chamberlain Group is a global leader in intelligent access and Blackstone portfolio company. Our innovative products, combined with intuitive software solutions, comprise a myQ ecosystem that delivers seamless, secure access to people’s homes and businesses. CG’s recognizable brands, including LiftMaster® and Chamberlain®, are found in 50+ million homes, and 11+ million people rely on our myQ® app daily to control and monitor their homes, communities and businesses, from anywhere. Our patented vehicle-to-home connectivity solution, myQ Connected Garage, is available in millions of vehicles from the leading automakers.
Media Contact:
Elise Houren
[email protected]
Thursday, October 3, 2024
Automatic Enrollment for Plumerai Familiar Face Identification!
We have launched Automatic Enrollment for our Plumerai Familiar Face Identification! Automatic Enrollment makes it effortless to register new individuals for identification; as soon as a person walks up to the camera, they will be automatically registered, and users can tag them with a name in the app.
This feature:
- identifies people from an 8m/26ft distance (often beyond),
- excels with a variety of camera positions, camera angles, and in low light conditions,
- and most importantly, it has been built to run on the device (not in the cloud!), so it doesn’t compromise on privacy.
Plumerai Familiar Face Identification is now operational on millions of cameras and we are proud to share that it is the most accurate solution for the smart home (outperforming Google Nest!). Offering this new feature really takes it to the next level.
Try our demo for yourself here and get in touch if you would like to utilize this incredible feature in your cameras.
Tuesday, September 24, 2024
High accuracy, even in the dark
Plumerai Familiar Face Identification enables smart home cameras to notify you when loved ones arrive safely, activate floodlights if a stranger loiters in your yard, and quickly search through recordings. It’s also a critical building block for the vision LLM features that we’re developing.
As shown in the video, it performs exceptionally well even in complete darkness, accurately and effortlessly identifying people. It works perfectly from many camera positions and with wide-angle lenses.
Our Plumerai Familiar Face Identification is already operational on millions of cameras. It’s the most accurate solution for the smart home (outperforming Google Nest!) and has been winning every commercial shootout.
Give our demo a try in your browser here!
Tuesday, August 27, 2024
Plumerai AI Factory
Developing accurate and efficient AI solutions involves a complex process where numerous tasks must work seamlessly together. We refer to this as our Plumerai AI factory.
Our AI factory is built on a foundation of carefully integrated components, each playing a crucial role in delivering accurate and robust AI solutions.
From data collection and labeling to model architecture and deployment, every step is meticulously designed to ensure optimal performance and accuracy. We continuously refine our algorithms and models, apply advanced training strategies, and conduct rigorous data unit tests to identify and mitigate potential failure cases.
This holistic approach enables us to deliver industry-leading AI technology that enhances the smart home experience, providing safety, convenience, and peace of mind for the end users.
Friday, July 26, 2024
Multi-camera smart homes are noisy!
Solve notification overload with Plumerai Re-ID.
Multi-camera smart homes enhance security, but more cameras mean more notifications. Imagine Emma arriving home to the house pictured below and walking through her home to the garden. Her household would receive three notifications in quick succession from the video doorbell, indoor camera, and outdoor camera. That’s not a good user experience!
At Plumerai, we solve this with our multi-camera Re-Identification AI, which recognizes individuals by their clothes and physique. It tracks the same person as they move around the home, reducing unnecessary alerts. When combined with our Familiar Face Identification, the notification can become even more specific. So, when Emma arrives home and moves through the house to the garden, users receive just one useful notification:
‘Emma arrived home.’
Thursday, July 18, 2024
Plumerai Re-ID groups video events
With Plumerai’s Re-identification AI embedded in your smart home cameras, they can remember and recognize a person’s clothing and physique. So, you can seamlessly merge video clips of the same individual into a single, comprehensive video. The result is a simplified, uncluttered activity feed, allowing users to quickly locate and view the video clips they’re interested in, without having to open multiple short clips.
Integrated with Plumerai’s Familiar Face Identification & Stranger Detection, the system also identifies known and unknown individuals. In the example below, users will receive just one, concise video of ‘Emma’ playing outside, instead of dozens of short videos.
Tuesday, July 9, 2024
Plumerai Package Detection AI
Integrate Plumerai Package Detection AI into your video doorbells and give your customers the knowledge of when and where their packages have been delivered. Our AI detects a wide variety of packages, their location, and drop off or pick up. Plumerai Package Detection is delivered as part of our full smart home camera AI solution.
Wednesday, June 26, 2024
Simply brilliant alerts with Plumerai Re-ID
Plumerai’s breakthrough Person Re-identification AI remembers and recognizes a person’s clothing and physique, enabling it to understand when the same person is going in and out of view. Combined with Plumerai’s Familiar Face & Stranger Identification, it can also recognize known and unknown individuals. So if Emma is gardening or taking out the garbage, household members receive a single, insightful alert rather than a flood of unhelpful notifications.
Thursday, June 20, 2024
Fisheye lens? No problem!
Fisheye lenses capture a much wider angle, so users can see a clear view of their porch. However, the fisheye distortion often makes accurate familiar face identification difficult. Plumerai’s AI has been designed to work with a wide variety of fields-of-view, enabling high detection accuracy even on wide angle lenses!
Tuesday, June 11, 2024
Plumerai Package Detection AI
Integrate Plumerai’s new Package Detection AI for video doorbells, so your customers know when packages are delivered to their home. Send snapshots of each package delivery or pickup to give them extra peace of mind.
Plumerai Package Detection AI integrates seamlessly with Plumerai People, Animal, Vehicle, and Stranger Detection, as well as Plumerai Familiar Face Identification.
Thursday, June 6, 2024
Accurate Stranger Detection AI
Plumerai is enhancing stranger detection! 🚀
Unlike other systems that flag ‘Stranger’ when no face match is found, our AI goes a step further. Plumerai’s technology can distinguish between unclear or distant faces and actual strangers. When the face is unclear, our algorithm labels it as ‘Identity Unknown’ rather than jumping to conclusions. As a result, you can rely our technology to power accurate notifications and security responses, such as turning on floodlights at night and activating alarms when you’re not at home.
Wednesday, April 17, 2024
Intelligent floodlights with Plumerai Stranger Detection AI
We’re taking smart home cameras to the next level with Plumerai’s Stranger Detection AI! Our technology accurately distinguishes between familiar faces and strangers, allowing your customers to tailor their alerts and security response. For an advanced smart home experience, link the doorbell to the home security system: welcome loved ones with warm lighting, and deter unwelcome visitors with red floodlights and an alarm.
Wednesday, March 20, 2024
No more "Did you get home ok?"
Offer peace of mind to your customers by deploying Plumerai’s Familiar Face Identification AI to your video doorbell. Our technology recognizes familiar faces, offering personalized alerts like “Jane arrived home”. The best part? Plumerai AI models run on the edge, so your customers’ images don’t leave the device, ensuring their privacy.
Try out our browser-based webcam demo here: plumerai.com/automatic-face-identification-live
Friday, December 15, 2023
Plumerai’s highly accurate People Detection and Familiar Face Identification AI are now available on the Renesas RA8D1 MCU
Discover the power of our highly efficient, on-device AI software, now available on the new Renesas RA8D1 MCU through our partnership with Renesas. In the video below, Plumerai’s Head of Product Marketing, Marco, showcases practical applications in the Smart Home and for IOT, and demonstrates our People Detection AI and Familiar Face Identification AI models.
The Plumerai People Detection AI fits on almost any camera with its tiny footprint of 1.5MB. It outperforms much larger models on accuracy, and achieves 13.6 frames per second on the RA8’s Arm Cortex-M85. Familiar Face Identification is a more complex task and requires multiple neural networks running in parallel, but still runs at 4 frames per second on the Arm Cortex-M85, providing rapid identifications.
All Plumerai models are trained on our diverse dataset of over 30 million images; our proprietary data tooling ensures that only good-quality and highly relevant data is utilized. Our models are proven in the field, and you can try them for yourself in your web browser:
Friday, November 24, 2023
Enable secure end-to-end encryption with Plumerai AI at the edge
To offer AI-powered alerts and recordings, smart home cameras typically send unencrypted footage to the cloud for AI analysis. This is because if the video is encrypted, the AI can’t analyze it; however, sending unencrypted data to the cloud poses risks for privacy and cyber attacks. With Plumerai, all the AI occurs on the camera, enabling you to offer end-to-end encryption on your smart home cameras, as well as advanced AI features! Plumerai gives you the best of both worlds without compromise.
Thursday, November 9, 2023
No more notification overload
Say goodbye to irrelevant notifications from your video doorbell. No more notifications from moving branches, shadows, or of yourself coming home. Plumerai’s technology enables your video doorbells to accurately detect people & vehicles and even recognizes household members. So you are only alerted about the events that matter to you.
Try it in your browser now: plumerai.com/automatic-face-identification-live
Thursday, October 26, 2023
Welcoming familiar faces. Keeping watch for strangers.
Upgrade your smart home cameras with Plumerai’s Familiar Face Identification AI for fewer, more relevant notifications. Our AI model runs inside the camera, so images don’t leave the device, protecting your customer’s privacy. Simply deploy our technology to your existing devices via an over-the-air software update.
Try our live AI demo in your browser: plumerai.com/automatic-face-identification-live
Monday, October 2, 2023
Arm Tech Talk: Accelerating People Detection with Arm Helium vector extensions
Watch Cedric Nugteren showcase Plumerai’s People Detection on an Arm Cortex-M85 with Helium vector extensions, running at a blazing 13 FPS with a 3.7x speed-up over Cortex-M7.
Cedric delves deep into Helium MVE, providing a comprehensive comparison to the traditional Cortex-M instruction set. He also demonstrates Helium code for 8-bit integer matrix multiplications, the core of deep learning models.
He shows a live demo on a Renesas board featuring an Arm Cortex-M85, along with a preview of the Arm Ethos-U accelerator, ramping up the frame rate even further to 83 FPS. Additionally, he showcases various other Helium-accelerated AI applications developed by Plumerai.
Monday, July 17, 2023
tinyML EMEA 2023: Familiar Face Identification
Imagine a TV that shows tailored recommendations and adjusts the volume for each viewer, or a video doorbell that notifies you when a stranger is at the door. A coffee machine that knows exactly what you want so you only have to confirm. A car that adjusts the seat as soon as you get in, because it knows who you are. All of this and more is possible with Familiar Face Identification, a technology that enables devices to recognize their users and personalize their settings accordingly.
Unfortunately, common methods for Familiar Face Identification are either inaccurate or require running large models in the cloud, resulting in high cost and substantial energy consumption. Moreover, the transmission of facial images from edge devices to the cloud entails inherent risks in terms of security and privacy.
At Plumerai, we are on a mission to make AI tiny. We have recently succeeded in bringing Familiar Face Identification to the edge. This makes it possible to identify users entirely locally — and therefore securely, using very little energy and with very low-cost hardware.
Our solution uses an end-to-end deep learning approach that consists of three neural networks: one for object detection, one for face representation, and one for face matching. We have applied various advanced model design, compression, and training techniques to make these networks fit within the hardware constraints of small CPUs, while retaining excellent accuracy.
In his talk at the tinyML EMEA Innovation Forum, Tim de Bruin presented the techniques we used to make our Familiar Face Identification solution small and accurate. By enabling face identification to run entirely on the edge, our solution opens up new possibilities for user-friendly and privacy-preserving applications on tiny devices.
These techniques are explained and demonstrated using our live web demo, which you can try for yourself right in the browser.
Tuesday, June 27, 2023
Plumerai wins MLPerf Tiny 1.1 AI benchmark for microcontrollers again
New results show Plumerai leads performance on all Cortex-M platforms, now also on M0/M0+
Last year we presented our MLPerf Tiny 0.7 and MLPerf Tiny 1.0 benchmark scores, showing that our inference engine runs your AI models faster than any other tool. Today, MLPerf released new Tiny 1.1 scores and Plumerai has done it again: we still lead the performance charts. A faster inference engine means that you can run larger and more accurate AI models, go into sleep mode earlier to save power, and/or run AI models on smaller and lower cost hardware.
The Plumerai Inference Engine compiles any AI model into an optimized library and runtime that executes that model on a CPU. Our inference engine executes the AI model as-is: it does no additional quantization, no binarization, no pruning, and no model compression. Therefore, there is no accuracy loss. The AI model simply runs faster than other solutions and has a smaller memory footprint. We license the Plumerai Inference Engine to semiconductor manufacturers such that they can include it in their SDK and make it available to their customers. In addition, we license the Plumerai Inference Engine directly to AI software developers.
Here’s the overview of the MLPerf Tiny 1.1 results for the STM32 Nucleo-L4R5ZI board with an Arm Cortex-M4 CPU running at 120MHz:
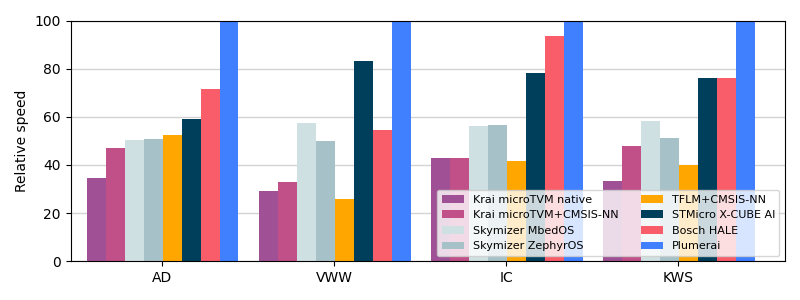
For comparison purposes, we also included TensorFlow Lite for Microcontrollers (TFLM) with Arm’s CMSIS-NN in orange, although this is not part of the official MLPerf Tiny results. To be clear: all results in the above graph compare inference engine software-only solutions. The same models run on the same hardware with the same model accuracy.
Since MLPerf Tiny 1.0, we have also improved our inference engine significantly in other areas. We have added new features such as INT16 support (e.g. for audio models), support for more layers, lowered RAM usage further, and added support for more types of microcontrollers.
We recently also extended support for the Arm Cortex-M0/M0+ family. Even though our results for these tiny microcontrollers are not included in MLPerf Tiny 1.1, we did run extra benchmarks with the official MLPerf Tiny software specifically for this blog post and guess what: we beat the competition again! In the following graph we present results on the STM32 Nucleo-G0B1RE board with a 64MHz Arm Cortex-M0+:
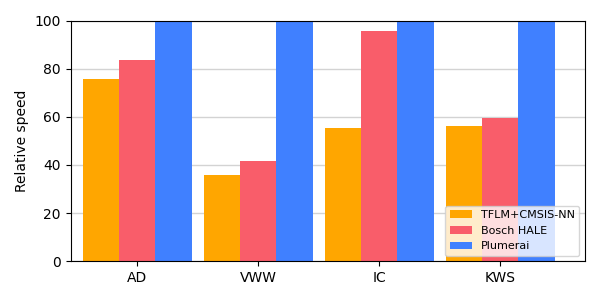
As mentioned before, our inference engine also does very well on reducing RAM usage and code size, which are often very important factors for microcontrollers due to their limited on-chip memory sizes. MLPerf Tiny does not measure those metrics, but we do present them here. The following table shows the peak RAM numbers for our inference engine compared to TensorFlow Lite for Microcontrollers (TFLM) with Arm’s CMSIS-NN:
Memory | Visual Wake Words | Image Classification | Keyword Spotting | Anomaly Detection | Reduction |
---|---|---|---|---|---|
98.80 | 54.10 | 23.90 | 2.60 | ||
36.60 | 37.90 | 17.10 | 1.00 | ||
2.70x | 1.43x | 1.40x | 2.60x | 2.03x |
And here are our code size results (excluding model weights) compared to TensorFlow Lite for Microcontrollers (TFLM):
Code size | Visual Wake Words | Image Classification | Keyword Spotting | Anomaly Detection | Reduction |
---|---|---|---|---|---|
187.30 | 93.60 | 104.00 | 42.60 | ||
54.00 | 22.50 | 20.20 | 28.40 | ||
3.47x | 4.16x | 5.15x | 1.50x | 3.57x |
Want to see how fast your models can run? You can submit them for free on our Plumerai Benchmark service. We compile your model, run it on a microcontroller, and email you the results in minutes. Curious to know more? You can browse our documentation online. Contact us if you want to include the Plumerai Inference Engine in your SDK.
Monday, March 13, 2023
World’s fastest MCU: Renesas runs Plumerai People Detection on Arm Cortex-M85 with Helium
Live demo at the Renesas booth at Embedded World from March 14-16
Plumerai delivers a complete software solution for people detection. Our AI models are fast, highly accurate, and tiny. They even run on microcontrollers. Plumerai People Detection and the Plumerai Inference Engine have now been optimized for the Arm Cortex-M85 also, including extensive optimizations for the Helium vectorized instructions. We worked on this together with Renesas, an industry leader in microcontrollers. Renesas will show our people detection running on an MCU that is based on the Arm Cortex-M85 processor at the Embedded World conference this week.
Microcontrollers are an ideal choice for many applications: they are highly cost-effective and consume very little power, which enables new battery-powered applications. They are small in size, and require minimal external components, such that they can be integrated into small systems.
Plumerai People Detection runs with an incredible 13 fps on the Renesas Helium-enabled Arm Cortex-M85, greatly outperforming any other MCU solution. It speeds up the processing of Plumerai People Detection by roughly 4x compared to an Arm Cortex-M7 at the same frequency. The combination of Plumerai’s efficient software with Renesas’ powerful MCU opens up new applications such as battery-powered AI cameras that can be deployed anywhere.
Faster AI processing is crucial for battery-powered devices because it allows the system to go to sleep sooner and conserve power. Faster processing also results in higher detection accuracy, because the MCU can run larger AI models. Additionally, a high frame rate enables people tracking to help understand when people are loitering, enter specific areas, or to count them.
Renesas has quickly become a leader in the Arm MCU market, offering a feature rich family of over 250 different MCUs. Renesas will implement the new Arm processor within its RA (Renesas Advanced) Family of MCUs.
Contact us for more information about our AI solutions and to receive a demo for evaluation.
Monday, March 6, 2023
Plumerai People Detection AI now runs on Espressif ESP32-S3 MCU
Plumerai People Detection AI is now available on Espressif’s ESP32-S3 microcontroller! Trained with more than 30 million images, Plumerai’s AI detects each person in view, even if partially occluded, and tracks up to 20 people across frames. Running the Plumerai People Detection on Espressif’s MCU enables new smart home, smart building, smart city, and smart health applications. Tiny smart home cameras based on the ESP32-S3 can provide notifications when people are on your property or in your home. Lights can turn on when we get home and the AC can direct the cold airflow toward you. The elderly can stay independent longer with sensors that notice when they need help. Traffic lights notice automatically when you arrive. In retail, customers can be counted for footfall analysis, and displays can show more detailed content when customers get closer to them. The Plumerai People Detection software supports indoor, outdoor, low light, and difficult backgrounds such as moving objects, and can detect at more than 20m / 65ft distance. The Plumerai People Detection runs completely at the edge and all computations are performed on the ESP32-S3. This means there is no internet connection needed, and the captured images never leave the device, increasing reliability and respecting privacy. In addition, performing the people detection task at the edge eliminates costly cloud compute. We are proud to offer a solution that enables more applications and products to benefit from our accurate people detection software.
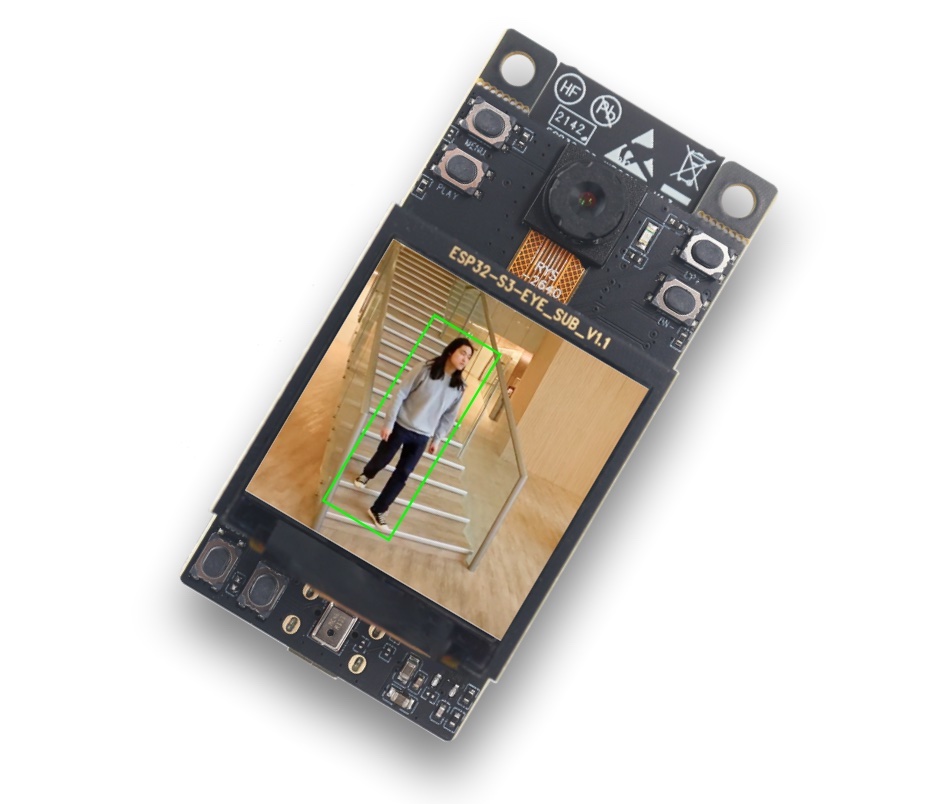
Resources required on the ESP32-S3 MCU:
- Single core Xtensa LX7 at 240 MHz
- Latency: 303 ms (3.3 fps)
- Peak RAM usage: 166 KiB
- Binary size: 1568 KiB
Demo is readily available:
- Runs on ESP32-S3-EYE board
- OV2640 camera, 66.5° FOV
- 1.3” LCD 240x240 SPI-based display
- Runs FreeRTOS
- USB powered
The ESP32-S3 is a dual-core XTensa LX7 MCU, capable of running at 240 MHz. Apart from its 512 KB of internal SRAM, it also comes with integrated 2.4 GHz, 802.11 b/g/n Wi-Fi and Bluetooth 5 (LE) connectivity that provides long-range support. We extensively optimized our people detection AI software to take advantage of the ESP32-S3’s custom vector instructions in the MCU, providing a significant speedup compared to using the standard CPU’s instruction set. Plumerai People Detection needs only one core to run at more than 3 fps, enabling fast response times. The second core on the ESP32-S3 is available for additional tasks.
A demo is available that runs on the ESP32-S3-EYE board. The board includes a 2-Megapixel camera, and an LCD display, which provides a live display of the camera feed and the bounding boxes, showing where and when people in view are detected.
The ESP32-S3-EYE board is readily available from many electronics distributors. To get access to the demo application fill in your email address and refer to the ESP32 demo. You can find more info on how to use the demo in the Plumerai Docs.
Plumerai People Detection software is available for licensing from Plumerai.
Wednesday, November 9, 2022
MLPerf Tiny 1.0 confirms: Plumerai’s inference engine is again the world’s fastest
Earlier this year in April we presented our MLPerf Tiny 0.7 benchmark scores, showing that our inference engine runs your AI models faster than any other tool. Today, MLPerf released the Tiny 1.0 scores and Plumerai has done it again: we still have the world’s fastest inference engine for Arm Cortex-M architectures. Faster inferencing means you can run larger and more accurate AI models, go into sleep mode earlier to save power, and run them on smaller and lower cost MCUs. Our inference engine executes the AI model as-is and does no additional quantization, no binarization, no pruning, and no model compression. There is no accuracy loss. It simply runs faster and in a smaller memory footprint.
Here’s the overview of the MLPerf Tiny results for an Arm Cortex-M4 MCU:
Vendor | Visual Wake Words | Image Classification | Keyword Spotting | Anomaly Detection |
---|---|---|---|---|
Plumerai | 208.6 ms | 173.2 ms | 71.7 ms | 5.6 ms |
STMicroelectronics | 230.5 ms | 226.9 ms | 75.1 ms | 7.6 ms |
301.2 ms | 389.5 ms | 99.8 ms | 8.6 ms | |
336.5 ms | 389.2 ms | 144.0 ms | 11.7 ms |
Compared to TensorFlow Lite for Microcontrollers with Arm’s CMSIS-NN optimized kernels, we run 1.74x faster:
Speed | Visual Wake Words | Image Classification | Keyword Spotting | Anomaly Detection | Speedup |
---|---|---|---|---|---|
335.97 ms | 376.08 ms | 100.72 ms | 8.45 ms | ||
194.36 ms | 170.42 ms | 66.32 ms | 5.59 ms | ||
1.73x | 2.21x | 1.52x | 1.51x | 1.74x |
But not only latency is important. Since MCUs often have very limited memory on board it’s important that the inference engine uses minimal memory while executing the neural network. MLPerf Tiny does not report numbers for memory usage, but here are the memory savings we can achieve on the benchmarks compared to TensorFlow Lite for Microcontrollers. On average we use less than half the memory:
Memory | Visual Wake Words | Image Classification | Keyword Spotting | Anomaly Detection | Reduction |
---|---|---|---|---|---|
98.80 | 54.10 | 23.90 | 2.60 | ||
36.50 | 37.80 | 17.00 | 1.00 | ||
2.71x | 1.43x | 1.41x | 2.60x | 2.04x |
MLPerf doesn’t report code size, so again we compare against TensorFlow Lite for Microcontrollers. The table below shows that we reduce code size on average by a factor of 2.18x. Using our inference engine means you can use MCUs that have significantly smaller flash size.
Code size | Visual Wake Words | Image Classification | Keyword Spotting | Anomaly Detection | Reduction |
---|---|---|---|---|---|
209.60 | 116.40 | 126.20 | 67.20 | ||
89.20 | 48.30 | 46.10 | 54.20 | ||
2.35x | 2.41x | 2.74x | 1.24x | 2.18x |
Want to see how fast your models can run? You can submit them for free on our Plumerai Benchmark service. We email you the results in minutes.
Are you deploying AI on microcontrollers? Let’s talk.
Wednesday, November 2, 2022
World’s fastest inference engine now supports LSTM-based recurrent neural networks
At Plumerai we enable our customers to perform increasingly complex AI tasks on tiny embedded hardware. We recently observed that more and more of such tasks are using recurrent neural networks (RNNs), in particular RNNs using the long-short-term-memory (LSTM) cell architecture. Example uses of LSTMs are analyzing time-series data coming from sensors like IMUs or microphones, human activity recognition for fitness and health monitoring, detecting if a machine will break down, and speech recognition. This led to us optimizing and extending our support for LSTMs and today we are proud to announce that Plumerai’s deep learning inference software greatly outperforms existing solutions for LSTMs on microcontrollers for all metrics: speed, accuracy, RAM usage, and code size.
To demonstrate this, we selected four common LSTM-based recurrent neural networks and measured latency and memory usage. In the table below, we compare our inference software against the September 2022 version of TensorFlow Lite for Microcontrollers (TFLM for short) with CMSIS-NN enabled. We choose TFLM because it is freely available and widely used. Note that ST’s X-CUBE-AI also supports LSTMs, but that only runs on ST chips and works with 32-bit floating-point, making it much slower.
These are the networks used for testing:
- A simple LSTM model from the TensorFlow Keras RNN guide.
- A weather prediction model that performs time series data forecasting using an LSTM followed by a fully-connected layer.
- A text generation model using a Shakespeare dataset using an LSTM-based RNN with a text-embedding layer and a fully-connected layer.
- A bi-directional LSTM that uses context from both directions of the ’time’ axis, using a total of four individual LSTM layers.
TFLM latency | Plumerai latency | TFLM RAM | Plumerai RAM | |
---|---|---|---|---|
Simple LSTM | 941.4 ms | 189.0 ms (5.0x faster) |
19.3 KiB | 14.3 KiB (1.4x lower) |
Weather prediction | 27.5 ms | 9.2 ms (3.0x faster) |
4.1 KiB | 1.8 KiB (2.3x lower) |
Text generation | 7366.0 ms | 1350.5 ms (5.5x faster) |
61.1 KiB | 51.6 KiB (1.2x lower) |
Bi-directional LSTM | 61.5 ms | 15.1 ms (4.1x faster) |
12.8 KiB | 2.5 KiB (5.1x lower) |
Board: STM32L4R9AI with an Arm Cortex-M4 at 120 MHz with 640 KiB RAM and 2 MiB flash. Similar results were obtained using an Arm Cortex-M7 board.
In the above table we report latency and RAM, which are the most important metrics for most users. The faster you can execute a model, the faster the system can go to sleep, saving power. Microcontrollers are also very memory constrained, making thrifty memory usage crucial. In many cases code size (ROM usage) is also important, and again there we outperform TFLM by a large margin. For example, Plumerai’s implementation of the weather prediction model uses 48 KiB including weights and support code, whereas TFLM uses 120 KiB.
The table above does not report accuracy, because accuracy is not changed by our inference engine. It performs the same computations as TFLM without extra quantization or pruning. Just like TFLM, our inference engine does internal LSTM computations in 16 bits instead of 8 bits to maintain accuracy.
In this blog post we highlight the LSTM feature of Plumerai’s inference software. However, other neural networks are also well supported, very fast, and low on RAM and ROM consumption, without losing accuracy. See our MobileNet blog post or the MLPerf blog post for examples and try out our inference software with your own model.
Besides Arm Cortex-M0/M0+/M4/M7/M33, we also optimize our inference software for Arm Cortex-A, ARC EM, and RISC-V architectures. Get in touch if you want to use the world’s fastest inference software with LSTM support on your embedded device.
Friday, September 30, 2022
Plumerai’s people detection powers Quividi’s audience measurement platform
We’re happy to report that Quividi adopted our people detection solution for its audience measurement platform. Quividi is a world leader in the domain of measuring audiences for digital displays and retail media. They have over 600 customers analyzing billions of shoppers every month, across tens of thousands of screens.
Quividi’s platform measures consumer engagement in all types of venues, outside, inside, and in-store. Shopping malls, vending machines, bus stops, kiosks, digital merchandising displays and retail media screens measure audience impressions, enabling monetization of the screens and leveraging shopper engagement data to drive sales up. The camera-based people detection is fully anonymous and compliant with privacy laws, since no images are recorded or transmitted.
With the integration of Plumerai’s people detection, Quividi expands the range of its platform capabilities, since our tiny and fast AI software runs seamlessly on any Arm Cortex-A processor, instead of on costly and power-hungry hardware. Building a tiny and accurate people detection solution takes time: we collect and curate our own data, design and train our own model architectures with over 30 million images, and then run them on off-the-shelf Arm CPUs using our world’s fastest inference engine software.
Besides measuring audiences, people detection can really enhance the user experience in many other markets: tiny smart cameras that alert you when there is someone in your backyard, webcams that ensure you’re always optimally in view, or air conditioners and smart lights that turn off automatically when you leave the room are just a few examples.
More information on our people detection can be found here.
Contact us to evaluate our solution and learn how to integrate it in your product.
Friday, May 13, 2022
tinyML Summit 2022: ‘Tiny models with big appetites: cultivating the perfect data diet'
Although lots of research effort goes into developing small model architectures for computer vision, real gains cannot be made without focusing on the data pipeline. We already mentioned the importance of quality data and some pitfalls of public datasets in an earlier blog post, and have been further improving our data tooling a lot since then to make our data processes even more powerful. We have incorporated several machine learning techniques that enable us to curate our datasets at deep learning scale, for example by identifying images that contributed strongly to a specific false prediction during training.
In this recording of his talk at tinyML Summit 2022, Jelmer demonstrates how our fully in-house data tooling leverages these techniques to curate millions of images, and describes several other essential parts of our model and data pipelines. Together, these allow us to build accurate person detection models that run on the tiniest edge devices.
Friday, May 6, 2022
MLPerf Tiny benchmark shows Plumerai's inference engine on top for Cortex-M
We recently announced Plumerai’s participation in MLPerf Tiny, the best-known public benchmark suite for evaluation of machine learning inference tools and methods. In the latest v0.7 of the MLPerf Tiny results, we participated along with 7 other companies. The published results confirm the claims that we made earlier on our blog: our inference engine is indeed the world’s fastest on Arm Cortex-M microcontrollers. This has now been validated and tested using standardized methods and reviewed by third parties. And what’s more, everything was also externally certified for correctness by evaluating model accuracy on four representative neural networks and applications from the domain: anomaly detection, image classification, keyword spotting, and visual wake words. In addition, our inference engine is also very memory efficient and works well on Cortex-M devices from all major vendors.
Visual Wake Words | Image Classification | Keyword Spotting | Anomaly Detection | |
---|---|---|---|---|
STM32 L4R5 | 220 ms | 185 ms | 73 ms | 5.9 ms |
STM32 F746 | 59 ms | 65 ms | 19 ms | 2.4 ms |
200 ms | 203 ms | 64 ms | 6.8 ms |
Official MLPerf Tiny 0.7 inference results for Plumerai’s inference engine on 3 example devices
You can read more about Plumerai’s inference engine in another blog post, or try it out with your own models using our public benchmarking service. Do contact us if you are interested in the world’s fastest inference software and the most advanced AI on your embedded device, or if you want to know more about the MLPerf Tiny results.
Tuesday, January 4, 2022
tinyML Talk: Demoing the world’s fastest inference engine for Arm Cortex-M
We recently announced Plumerai’s inference engine for 8-bit deep learning models on Arm Cortex-M microcontrollers. We showed that it is the world’s most efficient on MobileNetV2, beating TensorFlow Lite for Microcontrollers with CMSIS-NN kernels by 40% in terms of latency and 49% in terms of RAM usage with no loss in accuracy. However, that was just on a single network and it might have been cherry-picked. This presentation shows a live demonstration of our new service that you can use to test your own 8-bit deep learning models with Plumerai’s inference engine. In this talk Cedric explains what we did to get these speedups and memory improvements and shows benchmarks for the most important publicly available neural network models.
Monday, January 3, 2022
People detection for building automation with Texas Instruments
We’ve partnered with Texas Instruments. Together we’ll enable many more AI applications on tiny microcontrollers. Plumerai’s highly accurate people detection runs on TI’s tiny SimpleLink Wi-Fi CC3220SF MCU. Resource utilization is minimal: peak RAM usage is 170 kB, program binary size is 154 kB, and the detection runs in real-time on the low power Arm Cortex-M4 CPU at 80MHz. We’re controlling the lights here, resulting in a much better user experience. The product was demonstrated at CES 2022.
Thursday, December 9, 2021
Squeezing data center AI into a tiny microcontroller
How we replaced a $10,000 GPU with a $3 MCU, while reaching EfficientDet-level accuracy.
What’s the point of making deep learning tiny if the resulting system becomes unreliable and misses key detections or generates false positives? We like our systems to be tiny, but don’t want to compromise on detection accuracy. Something’s got to give, right? Not necessarily. Our person detection model fits on a tiny Arm Cortex-M7 microcontroller and is as accurate as Google’s much larger EfficientDet-D4 model running on a 250 Watt NVIDIA GPU.
Person detection
Whether it’s a doorbell that detects someone walking up to your door, a thermostat that stops heating when you go to bed, or a home audio system where music follows you from room to room, there are many applications where person detection greatly enhances the user experience. When expanded beyond the home, this technology can be applied in smart cities, stores, offices, and industry. To bring such people-aware applications to market, we need systems that are small, inexpensive, battery-powered, and keep the images at the edge for better privacy and security. Microcontrollers are an ideal platform, but they don’t have powerful compute capabilities, nor much memory. Plumerai takes a full system-level approach to bring AI to such a constrained platform: we collect our own data, use our own data pipeline, and develop our own models and inference engine. The end result is real-time person detection on a tiny Arm Cortex-M7 microcontroller. Quite an achievement to get deep learning running on such a small device.
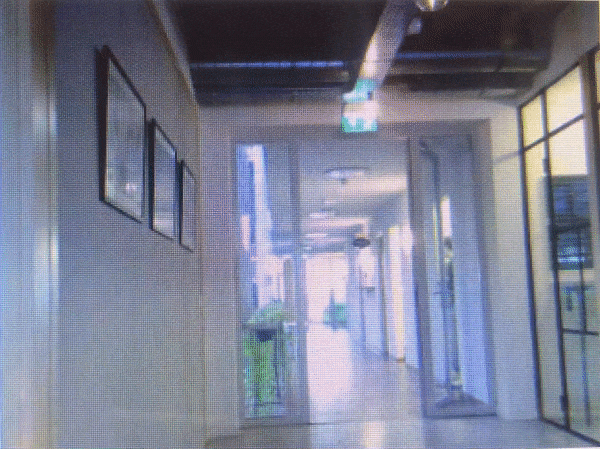
But what about accuracy?
Our customers keep asking this question too. Unfortunately, measuring accuracy is not that simple. A common approach is to measure accuracy on a public dataset, such as COCO. We have found this to be flawed, as many datasets are not accurate representations of real-world applications. For example, a model that performs better on COCO does not perform better in the field. This is because the dataset includes many images that are not relevant for a real-world use-case. In addition, there’s bias in COCO, as it was crowd-sourced from the internet. These images are mostly of situations that people found interesting to capture while always-on intelligent cameras mostly take pictures of uninteresting office hallways or living rooms. For example, the dark images in COCO include many from concerts with many people in view where a dark office or home is usually empty. The only right dataset to measure accuracy on is a relevant and clean dataset that is specifically collected for the target application. We do extensive, proprietary data collection and curation and decide to use our own test dataset to measure accuracy on. It goes without saying that this test dataset is completely independent and separate from the training dataset — collected in different locations with different backgrounds, situations, and subjects.
After selecting the dataset to measure accuracy on, we need to choose a model to compare to. We found Google’s state-of-the-art EfficientDet the most appropriate model for evaluation. EfficientDet is a large and highly accurate model that is scalable, enabling a range of performance points. EfficientDet starts with D0, which has a model size of 15.6MB, and goes up to D7x, which requires 308MB.
The surprising result? Our model is just as accurate as the pre-trained EfficientDet-D4. Both achieve an mAP of 88.2 on our test dataset. D4 has a model size of 79.7MB and typically runs on a $10,000 NVIDIA Tesla V100 GPU in a data center. Our model size is just 737KB, making it 112 times smaller. Using our optimized inference engine, our model runs in real-time on a tiny MCU with less than 256KB of on-chip RAM.
Model | Plumerai Person Detection | Google EfficientDet‑D4 |
---|---|---|
Model size | 737 KB | 79.7 MB |
Hardware | ArmCortex-M7 MCU | NVIDIA Tesla V100 GPU |
Price | <$3 | $10,000 |
Real-world person detection mAP | 88.2 | 88.2 |
Full stack optimization
Such a result can only be achieved with a relentless focus on the full AI stack.
First, choose your data wisely. A model is only as good as the data you train it with. We do extensive data collection and use our intelligent data pipeline for curation, augmentation, oversampling strategies, model debugging, data unit tests, and more.
Second, design and train the right model. Our model supports a single class, where EfficientDet can detect many. But such a multi-class model is not needed for our target application. In addition, we include our Binarized Neural Networks technology, significantly shrinking the network while also speeding it up.
Third, use a great inference engine. We developed a new inference engine from scratch, which greatly outperforms TensorFlow Lite for Microcontrollers. This inference engine works for both standard 8-bit as well as Binarized Neural Networks and does not affect accuracy.
Famous mathematician Blaise Pascal once said, “If I had more time, I would have written a shorter letter.” In deep learning, this translates to “If I had more time, I would have made a smaller model.” We did take that time, and it wasn’t easy, but we did build that smaller model. We’re excited to bring powerful deep learning to tiny devices that lack the storage capacity and the computational power for long letters.
Let us know if you’d like to continue the conversation. High accuracy numbers are nice, but nothing goes beyond running the model in the field. We’re always happy to jump on a call and show you our live demonstrations and discuss how we can bring AI to your products.
Monday, October 4, 2021
The world’s fastest deep learning inference software for Arm Cortex-M
New: Latest official MLPerf results are in!
New: Try out our inference engine with your own model!
At Plumerai we enable our customers to perform increasingly complex AI tasks on tiny embedded hardware. We’re proud to announce that our inference software for Arm Cortex-M microcontrollers is the fastest and most memory-efficient in the world, for both Binarized Neural Networks and for 8-bit deep learning models. Our inference software is an essential component of our solution, since it directs resource management akin to an operating system. It has 40% lower latency and requires 49% less RAM than TensorFlow Lite for Microcontrollers with Arm’s CMSIS-NN kernels while retaining the same accuracy. It also outperforms any other deep learning inference software for Arm Cortex-M:
Inference time | RAM usage | |
---|---|---|
TensorFlow Lite for Microcontrollers 2.5 (with CMSIS-NN) | 129 ms | 155 KiB |
Edge Impulse’s EON | 120 ms | 153 KiB |
MIT’s TinyEngine 1 | 124 ms | 98 KiB |
STMicroelectronics’ X-CUBE-AI | 103 ms | 109 KiB |
77 ms | 80 KiB |
Model: MobileNetV2 2 3 (alpha=0.30
, resolution=80x80
, classes=1000
)
Board: STM32F746G-Discovery at 216 MHz with 320 KiB RAM and 1 MiB flash
Our inference software builds upon TensorFlow Lite for Microcontrollers, such that it supports all of the same operations and more. But since resources are scarce on a microcontroller, we do not rely on TensorFlow or Arm’s kernels for the most performance-critical layers. Instead, for those layer types we developed custom kernel code, optimized for lowest latency and memory usage. This includes optimized code for regular convolutions, depthwise convolutions, fully-connected layers, various pooling layers and more. To become faster than the already heavily optimized Arm Cortex-M specific CMSIS-NN kernels, we had to go deep inside the inner-loops and also rethink the higher-level algorithms. This includes optimizations such as hand-written assembly blocks, improved register usage, pre-processing of weights and input activations and template-based loop-unrolling.
Although these generic per-layer-type optimizations resulted in great speed-ups, we went further and squeezed out every last bit of performance from the Arm Cortex-M microcontroller. To do that, we perform specific optimizations for each layer in a neural network. For instance, rather than only optimizing convolutions in general, our inference software makes specific improvements based on all actual values of layer parameters such as kernel sizes, strides, padding, etc. Since we do not know upfront which neural networks our inference software might run, we make these optimizations together with the compiler. This is achieved by generating code in an automated pre-processing step using the neural network as input. We then guide the compiler to do all the necessary constant propagation, function inlining and loop unrolling to achieve the lowest possible latency.
Memory usage is an important constraint on embedded devices; however fast or slow the software is, it has to fit in memory to run at all. TensorFlow Lite for Microcontrollers already comes with a memory planner that ensures a tensor only takes up space while there is a layer using it. We further optimized memory usage with a smart offline memory planner that analyzes the memory access patterns of each layer of the network. Depending on properties such as filter size, the memory planner allows the input and output of a layer to partially or even completely overlap, effectively computing the layer in-place.
Besides Arm Cortex-M, we also optimize our inference software for Arm Cortex-A and RISC-V architectures. And if the above results are still not fast enough for your application, we go even further. We make our AI tiny and radically more efficient by using Binarized Neural Networks (BNNs) - deep learning models that use only a single bit to encode each weight and activation. We are building improved deep learning model architectures and training algorithms for BNNs, we are designing a custom IP-core for customers with FPGAs and we are composing optimized training datasets. All these improvements mean that we can process more frames per second, save more energy, run larger and more accurate AI models and deploy on cheaper hardware.
Get in touch if you want to use the world’s fastest inference software and the most advanced AI on your embedded device.
Wednesday, October 13, 2021: Updated memory usage to match newest version of the inference software.
-
Results copied from https://github.com/mit-han-lab/tinyml/tree/master/mcunet, all other results were measured by us. ↩︎
-
The explicit padding layers in MobileNetV2 were fused, and to be able to compare with the TinyEngine results the number of filters in the final convolution layer (1280) was scaled by alpha to 384. The exact model can be downloaded here. ↩︎
-
microTVM ran out of memory, other benchmarks show that microTVM is generally a bit slower than CMSIS-NN. ↩︎
Tuesday, August 17, 2021
Great TinyML needs high-quality data
So far we have mostly written about how we enable AI applications on tiny hardware by using Binarized Neural Networks (BNNs). The use of BNNs helps us to reduce the required memory, the inference latency and energy consumption of our AI models, but there is something that we have been less vocal about that is at least as important for AI in the real world: high-quality training data.
To train tiny models, choose your data wisely
Deep learning models are famously hungry for training data, as more training data is usually the most effective way to improve accuracy. But once we started to train deep learning models that are truly tiny — with model sizes of a few hundred KB or less for computer vision tasks like person detection — we discovered that it is not so much the quantity but the quality of the training data that matters.
The key insight is that these tiny deep learning models have limited information capacity, so you cannot afford to waste precious KBs on learning irrelevant features. You have to be very strict in telling the model what you do and do not find important. We can communicate this to the model by carefully selecting its training data. Furthermore, the balance in your dataset is important because a compressed model tends to limit itself to perform well only on the concepts for which there are many samples in the training dataset. So we curate our datasets to ensure that all the important use-cases are included, and in the right balance.
Know your model
As tiny AI models become a part of our world, it is crucial that we know these models well. We have to understand in what situations they are reliable and where their pitfalls are.
High-level metrics like accuracy, precision and recall don’t provide anything close to the level of detail required here. Instead — taking inspiration from Andrej Karpathy’s Software 2.0 essay — we test our models in very specific situations. And rather than writing code we express these tests with data. Every model that we ship needs to surpass an accuracy threshold for very specific subsets of our dataset. For example, for person detection we have implemented tests for people standing far away or for people who are only showing their back, and for scenes containing coats and other fabrics that might confuse the model.
Several samples from our
person_standing_back
data unit test.
These data unit tests guarantee that the model will work reliably for our customers’ use-cases and also enable us to ensure our models are not making mistakes due to fairness-related attributes, such as skin color or gender. As we experiment with new training algorithms, model architectures and training datasets, our data unit tests allow us to track the progress these inventions make on the trained models that we ship to customers.
The outcome of some of our data unit tests — a standard MobileNet person detection model (left) versus Plumerai’s person detection model (right). The
person_hallway_beyond_7m
test result shows us that this model can not yet be reliably used to detect people from large distances.
Public datasets: handle with care
Public datasets are usually composed of photos taken by people for the enjoyment of people, such as photos of concerts, food or art. These photos are typically very different from the scenes in which TinyML/low-power AI products are deployed. For example, the dark photos in public datasets are mostly from concerts and almost always have people in them. So if this dataset is used to train a tiny deep learning model, the model will try to take a shortcut and associate dark images with the presence of people. This hack works fine as long as the model is evaluated on the same distribution of images, as is the case in the validation sets of public datasets, but will cause problems once the model is deployed in a smart doorbell camera. In addition, public datasets often contain misclassified images, images encoding harmful correlations and images that although technically labelled correctly are too difficult to classify correctly.
Most dark photos in the public Open Images dataset are from concerts and contain people. A tiny deep learning model trained on this dataset will try to take a problematic shortcut and associate dark images with the presence of people.
To circumvent the many problems of public datasets, we at Plumerai collect our own data — straight from the cameras used in TinyML products in the situations that our products are intended for. But that does not mean that we do not use any public datasets to train our models. We want to benefit from the scale and diversity of these datasets, while mitigating their quality issues. So we use our own data and analysis methods to automatically identify specific issues stemming from sampling biases in the public datasets and solve those problematic correlations that our models are sensitive to.
The images in public person detection datasets (left) are largely irrelevant to the scenes encountered by TinyML devices in the real-world (right).
One of the tools we can use to debug our tiny AI models are saliency maps. They allow us to see what our models are correctly (left) or incorrectly (right) triggered by.
Building the tiny AI model factory
Although new training optimizers and new model architectures get most of the attention, there are many other components required to build great AI applications on tiny hardware. Data unit tests and tools for dataset curation are some of the components that are mentioned above, but there are many more. We build tools to identify what data needs to be labelled, use large models in the cloud for auto labeling our datasets and combine these with human labelling. We build the whole infrastructure that is necessary to go as fast as possible through our model development cycle: train a tiny AI model, test it, identify the failure cases, collect and label data for those failure cases and then go through this cycle again and again. All these components together allow us to build tiny but highly accurate AI models for the real world.
ML code is just one small component of the large and complex tiny AI model factory that we are building. From Sculley et al. (2015).
Thursday, July 1, 2021
BNNs for TinyML: performance beyond accuracy — CVPR 2021 Workshop on Binarized Neural Networks
Tim de Bruin, a Deep Learning Scientist at Plumerai, was one of the invited speakers at last week’s CVPR 2021 Workshop on Binarized Neural Networks for Computer Vision. Tim presented some of Plumerai’s work on solving the remaining challenges with BNNs and explains why optimizing for accuracy is not enough.
Tuesday, April 20, 2021
tinyML Summit 2021: Person Detection under Extreme Constraints — Lessons from the Field
At this year’s tinyML Summit, we presented our new solution for person detection with bounding boxes. We have developed a person detection model that runs in real time (895 ms latency) on an STM32H7B3 board (Arm Cortex-M7), a popular off-the-shelf available microcontroller. To the best of our knowledge, this is the first time anyone runs person detection in real-time on Arm Cortex-M based microcontrollers, and we are very excited to be bringing this new capability to customers!
Watch the video below to see the live demo and learn how Binarized Neural Networks are an integral part of our solution.
Wednesday, April 7, 2021
MLSys 2021: Design, Benchmark, and Deploy Binarized Neural Networks with Larq Compute Engine
We are very excited to present our paper Larq Compute Engine: Design, Benchmark, and Deploy State-of-the-Art Binarized Neural Networks at the MLSys 2021 conference this week!
Larq Compute Engine (LCE) is a state-of-the-art inference engine for Binarized Neural Networks (BNNs). LCE makes it possible for researchers to easily benchmark BNNs on mobile devices. Real latency benchmarks are essential for developing BNN architectures that actually run fast on device, and we hope LCE will help people to build even better BNNs.
In the paper, we discuss the design of LCE and go into the technical details behind the framework. LCE was designed for usability and performance. It extends TensorFlow Lite, which makes it possible to integrate binarized layers into any model supported by TFLite and run it with LCE. Integration with Larq makes it very easy to move from training to deployment. And thanks to our hand-optimized inference kernels and sophisticated MLIR-based graph optimizations, inference speed is phenomenal.
The impact of binarization on the latency of different convolutional blocks in ResNets - binary is up to 17x faster than 32-bit floating point and 12x faster than 8-bit integers on a Pixel 1 phone.
BNNs are all about efficient inference: their tiny memory footprint and compact bitwise computations make them perfect for edge applications and small, battery powered devices. However, this requires the people building BNNs to have access to real, empirical measurements of their model as it is deployed. Lacking the right tools, researchers too often refrain to proxy metrics such as the number of FLOPs. LCE aims to change this.
In the paper, we demonstrate the value of measuring latency directly by analyzing the execution of some of the best existing BNN designs, such as R2B and BinaryDenseNets. We identify suboptimal points in these models and present QuickNet, a new model family which outperforms all existing BNNs on ImageNet in terms of latency and accuracy.
Breakdown of the latency of QuickNet-Large (QNL), our new state-of-the-art BNN.
Note how compared to Real-to-Binary Net (R2B) and BinaryDenseNet, we win mostly on the high-precision first layer and ‘glue’ layers.
We will be presenting the work at the MLSys conference on Wednesday the 7th of April. The published paper is publicly available, and registered attendees will be able to view our oral talk. We hope you will all attend, and if you want to learn more about running BNNs on all sorts of devices, contact us at [email protected]!
Friday, January 22, 2021
tinyML Talks: Binarized Neural Networks on microcontrollers
For the past few months we have been working very hard on something new: Binarized Neural Networks on microcontrollers. By bringing deep learning to cheap, low-power microcontrollers we remove price and energy barriers and make it possible to embed AI into basically any device, even for relatively complex tasks such as person detection.
This week, we gave a presentation as part of the tinyML Talks webcast series where we explained what we had to build to make this work. We demonstrated how combining our custom training algorithms, inference software stack and datasets results in a highly accurate and efficient solution - in this case for person presence detection on the STM32L4R9, an ARM Cortex-M4 microcontroller from STMicroelectronics. This technology can be implemented to trigger push notifications for smart home cameras, wake up devices when a person is detected, detect occupancy of meeting rooms and for many more applications. This is a step towards our goal of making deep learning ultra low-power and a future where battery-powered peel-and-stick sensors can perform complex AI tasks everywhere.
Our BNN models with our proprietary inference software are faster and more accurate on ARM Cortex-M4 microcontrollers than the best publicly available 8-bit deep learning models with TensorFlow Lite for Microcontrollers.
We quickly found out that great training algorithms and inference software are not enough when building a solution for the real world. Collecting and labeling our own data turned out to be crucial in dealing with a wide variety of difficulties.
Testing our models thoroughly is equally important. Instead of relying only on simplistic metrics such as accuracy, we developed a suite of unit tests to ensure reliable performance in many settings.
Bringing all of this together results in a highly robust and efficient solution for person presence detection, as we showed in our live demo.
We’re just scratching the surface with person detection and we have a lot more in store for the coming months - more applications on Arm Cortex-M microcontrollers, and even better performance with our own IP-core for BNN inference on low-powered FPGAs and with the xcore.ai platform from XMOS.
We are very happy with the high attendance during the live webcast and a ton of questions were submitted during the Q&A. There was no time to answer all of them live, so we shared our answers on the tinyML forum and we’ll be sharing more of our progress at the tinyML Summit in March.
If you’re thinking about using Binarized Neural Networks to enable highly accurate deep learning on microcontrollers, get in touch!
Wednesday, April 1, 2020
XMOS and Plumerai partner to accelerate commercialisation of binarized neural networks
XMOS and Plumerai bring together their deep learning expertise across chip design and algorithms in a Binarized Neural Network capability, advancing the deployment of intelligence at the edge.
Bristol & London UK, 1 April 2020 – British technology companies XMOS and Plumerai have agreed a new strategic partnership that will support the development of binarized neural network (BNN) capabilities that enable AI to be embedded in a wide range of everyday devices efficiently at low-power and at low-cost.
The partnership will combine Plumerai’s Larq software library for training BNNs and the xcore.ai crossover processor from XMOS which provides native support for inference of BNNs. The combination of the two technologies will deliver a BNN capability that’s 2 to 4x more efficient than existing edge AI solutions.
This solution will enable a new generation of devices to run tasks that make our lives simpler and safer. This could include everything from identifying that a shopping package has been delivered to a safe place to managing traffic flows more efficiently, supporting remote healthcare applications or keeping shelves in stores stocked more efficiently. While BNNs are an emerging technology, the future potential is enormous.
The deep learning revolution is all around us today. But a typical application uses deep learning models with tens of millions of parameters – and despite the move to 16-bit and 8-bit encoding there is still an insatiable demand to increase the speed and efficiency of deep learning and AI systems. That’s where BNNs come in.
BNNs are the most efficient form of deep learning, offering to transform the economics and efficiency of edge intelligence by going all the way down to just a single bit. However, there are significant challenges involved in making BNNs commercially viable – for example, they demand specific attention in chip design for efficient inference and new software algorithms for training.
XMOS and Plumerai have combined their respective expertise in embedded chip design and deep learning algorithms to enable this breakthrough technology and bring AI to the devices all around us.
Mark Lippett, XMOS CEO says “BNNs gained prominence in the news recently with Apple’s purchase of Xnor.ai for a reported $200m. It’s little surprise that Apple is exploring AI capabilities at the edge, with advanced machine learning algorithms that can run efficiently in low-power, offline environments.
“Regardless of other moves in the market, our partnership with Plumerai is exciting for AI developers around the world. The combination of Larq and xcore.ai offers the first consolidated path to commercially deploying BNNs, which will be highly disruptive in intelligent embedded systems.”
Roeland Nusselder, Plumerai CEO adds “We are thrilled to join forces with the experienced team from XMOS to bring BNNs to the edge, and we share their excitement about the emerging era of intelligent connectivity. Binarized deep learning has tremendous potential for enabling a new generation of energy-efficient, AI-powered applications. Our two companies are perfectly positioned to turn this potential into reality.”
About XMOS
XMOS is a deep tech company at the leading edge of the AIoT. Since its inception in 2005, XMOS has had its finger on the pulse recognising and addressing the evolving market need. The company’s processors put intelligence, connectivity and enhanced computation at the core of smart products.
About Plumerai
Plumerai is making deep learning tiny and computationally radically more efficient to enable real-time inference on the edge – for automated warehouses, retail, smart cameras, micromobility and many more. The team is based in London, Amsterdam and Warsaw and is backed by world-class investors.
Tuesday, March 24, 2020
The Larq Ecosystem
State-of-the-art binarized neural networks and even faster inference
In our previous blog post, we announced Larq Compute Engine (LCE), our deployment solution for Binarized Neural Networks (BNNs). The combination of LCE, our training library Larq and the models in Larq Zoo forms the first end-to-end solution for anyone building applications using BNNs. In this post, we take a step back and look at this integrated ecosystem as a whole.
Monday, February 17, 2020
Announcing Larq Compute Engine v0.1
Optimized BNN inference for edge devices
We believe BNNs are the future of efficient inference, which is why we’ve developed tools to make it easier to train and research these models. Our open-source library Larq enables developers to build and train BNNs and integrates seamlessly with TensorFlow Keras. Larq Zoo provides implementations of major BNNs from the literature together with pretrained weights for state-of-the-art models.
But the ultimate goal of BNNs is to solve real-world problems on the edge. So once you’ve built and trained a BNN with Larq, how do you get it ready for efficient inference? Today, we’re introducing Larq Compute Engine to tackle that problem.